5 Requirements for Investing in AI Startups
“Artificial intelligence will be more profound for humanity than fire and electricity.” - Sundar Pichai (CEO - Alphabet/Google)
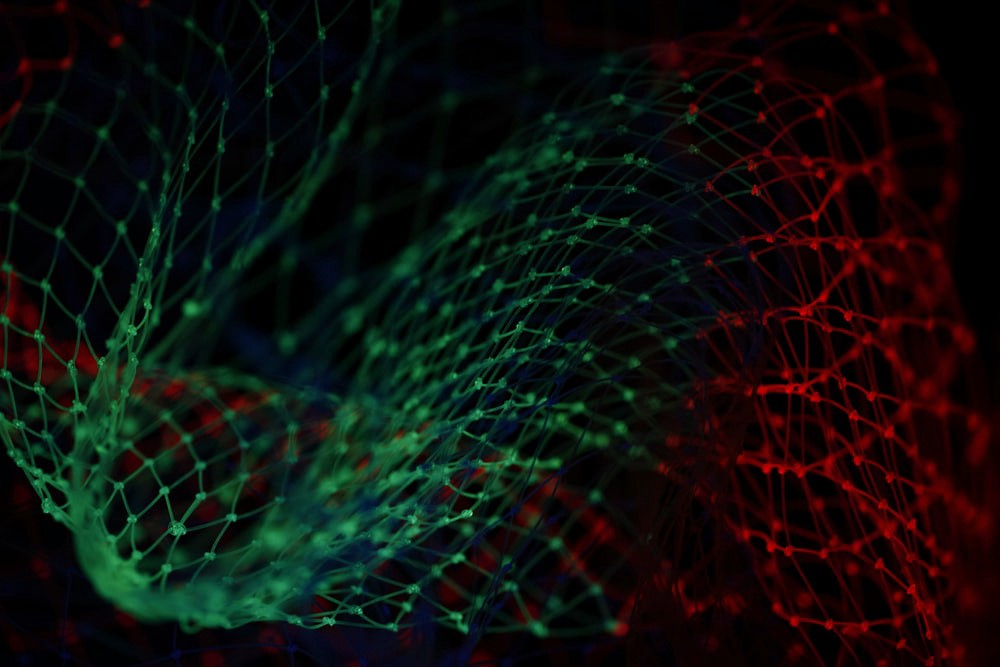
I believe that early-stage AI offers the most exciting investment opportunity of our generation. However, analysing these investments is not straightforward. Early-stage AI businesses are fundamentally different to their SaaS counterparts.
In many ways, they look the same — 2 or 3 scrappy founders in their early 30s… a polished pitch deck explaining how the business is solving a major problem in a large market… products that are built from code, live on cloud servers, have a sleek UI, and integrate with existing systems via APIs.
However, the economics of a SaaS startup are fundamentally different to that of an early-stage AI. The attraction of SaaS lies in the ability to build it once (at a relatively low fixed cost) and sell it an infinite number of times (at a negligible variable cost). Contrastingly, significant fixed costs are required to build and train the data models that are at the core of an AI. What’s more, these models are like an open fire — needing constant fuel (data and processing power) in order to avoid burning out (eg through data drift). As such, AI suffers from consistently high variable costs. These characteristics mean that to build a scalable AI business, one needs a very different playbook.
The following are 5 requirements that I have for early-stage (pre-seed to Series A) AI investments. I hope that they prove helpful to both investors and entrepreneurs who are active in this space.
Requirement 1: Laser focus 🔍
Early-stage AI should be more focussed on a specific problem and/or segment of the market than their SaaS counterparts.
There are two reasons for this:
Better economics
Most AI models are based on supervised learning. This requires collecting and processing vast amounts of clean and labelled data. The fixed costs associated with data acquisition can be considerable – particularly if this data is purchased from multiple sources. Once the data has been acquired, it must be cleaned and labelled, which is often a highly manual and labour intensive process. Finally, processing the data to train the model requires considerable cloud compute costs. These steps make AI costly to build and scale.
The amount of data that is needed by an AI model is correlated with the breadth of the problem that it is trying to solve. The broader the problem — the more edge cases that are likely to arise. As such, addressing a problem that is too broad will lead to excessive resource requirements.
By being laser focussed on (i) what the exact problem is; (ii) who the customers are; (iii) which data is needed, and (iv) what level of accuracy is optimal — a startup can optimise its cost base. The lesson, as Chris Dixon perfectly articulates it is: ‘Narrow the domain’ and then Narrow the domain even more’. You can always expand the scope later.
Whereas a SaaS business may be targeting the whole of market X, I would prefer an AI to target a subset of X initially — provided that subset’s revenue potential is large enough to yield venture returns (discussed further in requirement 2).
Note that this assumption may not hold true in a GPT-3 world, which would dramatically reduce the cost of data acquisition and model training. However, it is still too early to know with any certainty whether this will be the case.
Less competition
AI is synonymous with behemoths. Microsoft, Amazon, Facebook, IBM, Alphabet, Apple, Intel, Baidu and Alibaba have all invested heavily in the space. Generally speaking, these players are developing ‘horizontal AI’ solutions— creating AI that can be applied across multiple industries and use cases simultaneously (eg Siri or Alexa). They seek broader (and by implication more lucrative) market opportunities.
By opting for a more focussed approach, a startup can avoid direct competition with larger incumbents. Even in the face of direct competition, by focussing on a specific niche, a startup can create a competitive advantage by designing a product experience that is better catered to the customers within that specific domain.
Requirement 2: A large SAM (not just TAM) 📈
The economics of venture capital means that potential investments must have a vast Total Addressable Market (TAM). It is unlikely that most startups will be able to capture >10% of a market within the investment horizon (~10 years). As such, the market that is being captured must be large enough to create a reasonable chance that the business will some day be “venture scale” (>$100m in ARR).
However, investors also acknowledge that the best strategy for a startup is to initially focus on a niche within the broader market, achieve product-market fit, and subsequently expand into adjacent markets. This initial target market is known as the Serviceable Available Market (SAM).
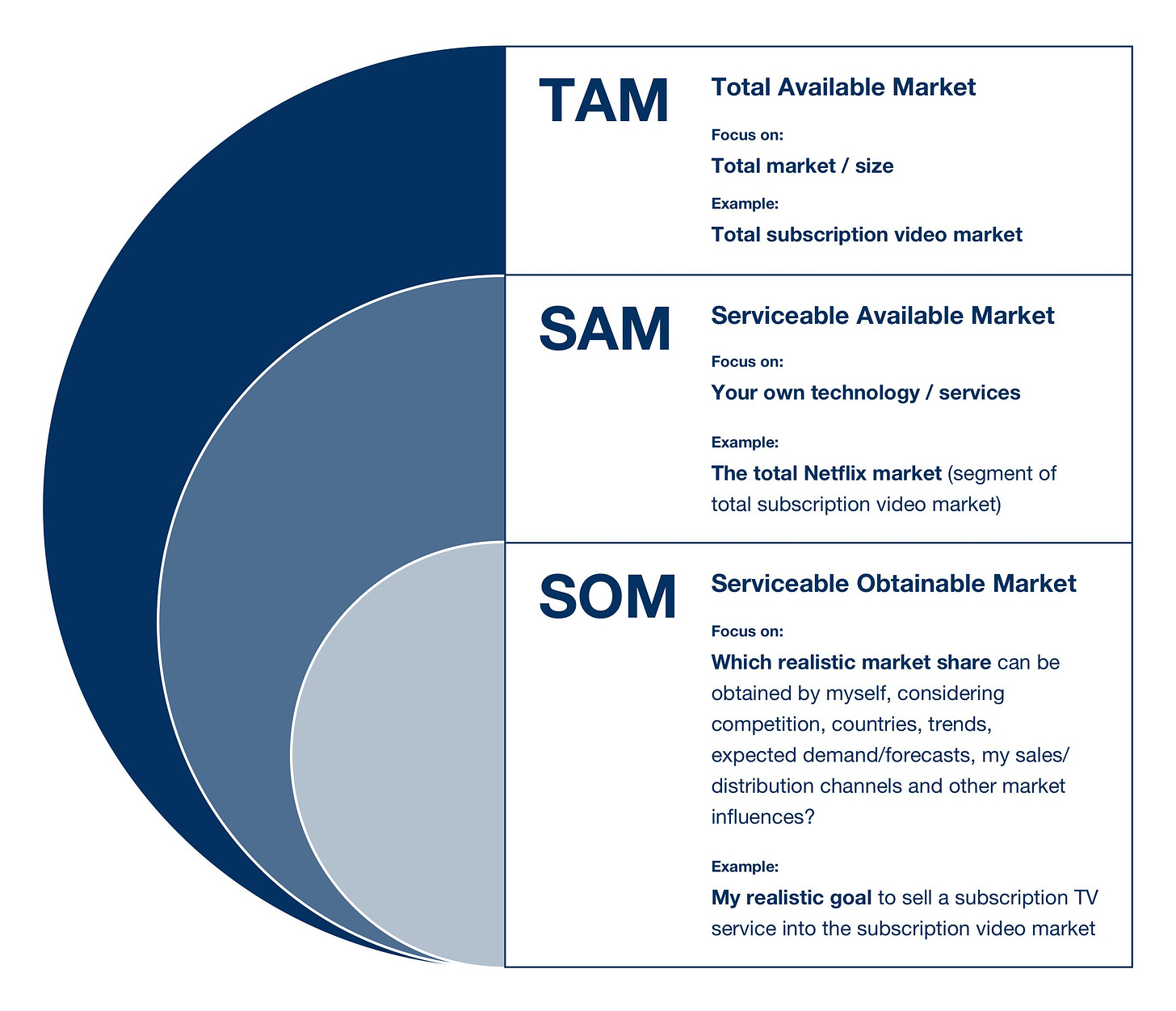
The nature of software development means that the cost of entering adjacent markets is very low — making it straight forward for SaaS startups to expand from their SAM to their TAM. Investors are willing to accept a lower SAM initially — with the expectation that the company can expand into new markets in the future.
Expanding into adjacent markets is much harder for an AI startup. AI models are not well suited to lateral thought (the ability to take experiences from one set of circumstances to a different set). A SaaS business can enter an adjacent market with only minimal dev and sales resource allocation. Contrastingly, an AI will need to be re-build and/or re-train their model to address the new customer problem. As discussed above, this is very costly. What’s more, addressing this new problem will likely require a new dataset — eroding any previous data defensibility that the company had built. These factors make moving from the SAM to the TAM very difficult — even if the use cases look similar on the surface.
As a result of this characteristic, I look for AI startups whose current product caters to a vast SAM — with the assumption that they will not be able to expand into adjacent markets in the near-term.
Requirement 3: Data defensibility 🛡
The best way for an AI startup to create a sustainable competitive advantage is through data. The algorithms underlying the model are less defensible, in my view. Many of these architectures are publicly available — having being built in academic settings. Pre-trained models can be accessed via open-source libraries — and model parameters can be optimised automatically.
As such, I believe that the best thing an AI startup can do is to create a data moat. I do not mean simply seeking to achieve scale. Indeed, I believe that data has limited scale advantages. Instead, I prefer a company to have (i) access to proprietary data; (ii) a unique process for combining and enriching data from publicly available sources; (iii) a process for gathering data in a scalable and cost effective way; (iv) a process for creating reliable synthetic data (eg using Generative adversarial networks).
Founders should be comfortable articulating why they have the optimal strategy with regards to data acquisition, processing, quality, storage and provisioning.
Requirement 4: High value use cases with a low risk of failure 💰
For AI startups to have commercial viability, they must be able to yield significant value for customers (through revenue generation or cost reduction).
Generally speaking, B2B AI integrations are more cumbersome relative to their SaaS equivalents. They require a notable time and resource commitment from potential customers. These customers will not be willing to devote these resources for a ‘nice to have’ product. Instead, the ROI of adopting the AI should make it a ‘must-have’ – where the cost-benefit trade off to the customer is clear (and preferably immediate). I look for use cases where AI can solve the problem 10x more effectively than non-AI alternatives.
However, the use cases must also have a low-risk of failure. The old AI adage is that AI is “really good at partially solving just about any problem.” Most startups can build a model that can achieve between 80–90% accuracy. However, it becomes exponentially harder to increase accuracy beyond this. The resource requirements to achieve >95% make the economics unfeasible for most use cases. As such, I prefer startups that cater to use cases where an approximation is sufficient.
Requirement 5: No need for explainability 🤷♂️
AI is superior to humans in many regards. For example, with regards to assignment (identifying what something is, or the extent to which items are connected); grouping (determining correlations and subsets in data); generation (creating images or text based on inputs) and forecasting (predicting changes in time series data).
However, one fundamental shortcoming of AI currently is its inability to explain why it has come to the conclusions that it has — known as the ‘black box dilemma’. AI models are trained with a lot of parameters on which transformations are applied. Subsequently, the process of pre-processing and model construction becomes a black-box that is very hard to interpret by the end user.
For example, in the context of decision making, AI can produce predictions about individuals’ credit risk and health status by mapping a user’s features into classes. However, the exact reasons for these connections are not elaborated. The prediction comes with no justification. More worryingly, in some cases, the algorithms have actually inherited human prejudices or biases that are hidden within the training data. This can result in situations where AI is producing unfair or even abhorrent decisions on critical issues.
A lot of work is being done on the development of Explainable AI (XAI). currently. However, we are still years away from achieving widespread XAI. I prefer an early-stage company to be addressing a problem where the absence of an explanation has only minor implications (ie content recommendations instead of “big ticket” decisions like military strategy or medical diagnoses).
Concluding thoughts
Early-stage AI founders have a more challenging road ahead of them relative to their SaaS equivalents. However, by truly understanding the economic characteristics of AI, they can build highly profitable businesses, that address some of the most important problems of our time.
If you’re an early-stage AI founder with a focus on video, audio or gaming — drop me a line on omar@rooksnestventures.com. I’ll respond! 👋
Nice write up!